News
FUSION 2019
Successful submissions from CRISP researchers of Fraunhofer IGD
CRISP researchers of Fraunhofer IGD place papers at Conferences 22nd International Conference on Information Fusion, FUSION. The International Conference on Information Fusion provides the best forum to present foundational, technological, and application-focused innovations in the sensor, data, information and knowledge fusion scientific domains.
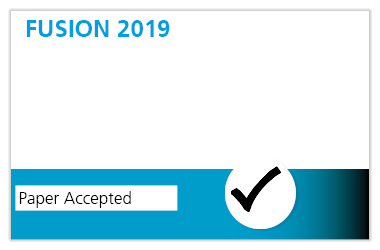
For more than two decades, this distinctive conference has brought together researchers and practitioners from academia, industry, and government agencies working in these domains with application to surveillance, aerospace, robotics, intelligent transportation, sensor networks and biomedical engineering, among others. The 2019 conference is focused on advancing the employment of multidisciplinary and innovative methods for solving the most challenging problems in the field, inclusive of methods that could be called disruptive to the traditional concepts in data and information fusion.
Accepted papers are:
A Multi-detector Solution Towards an Accurate and Generalized Detection of Face Morphing Attacks
Authors: Naser Damer, Steffen Zienert, Yaza Wainakh, Alexandra Moseguí Saladié, Florian Kirchbuchner, Arjan Kuijper (all Fraunhofer IGD)
Abstract: Face morphing attack images are built to be verifiable to multiple identities. Associating such images to identity documents leads to building faulty identity links, causing vulnerabilities in security critical processes. Recent works have studied the face morphing attack detection performance over variations in morphing approaches, pointing out low generalization. This work introduces a multi-detector fusion solution that aims at gaining both, accuracy and generalization over different morphing types. This is performed by fusing classification scores produced by detectors trained on databases with variations in morphing type and image pairing protocols. This work develop and evaluate the proposed solution along with baseline solutions by building a database with three different pairing protocols and two different morphing approaches. This proposed solution successfully lead to decreasing the Bona Fide Presentation Classification Error Rate at 1.0% Attack Presentation Classification Error Rate from 15.7% and 3.0% of the best performing single detector to 2.7% and 0.0%, respectively on two face morphing techniques, pointing out a highly generalized performance
Multi-algorithmic Fusion for Reliable Age and Gender Estimation from Face Images
Authors: Philipp Terhörst, Marco Huber, Jan Niklas Kolf, Naser Damer, Florian Kirchbuchner, Arjan Kuijper (all Fraunhofer IGD)
Abstract: Automated estimation of demographic attributes, such as gender and age, became of great importance for many potential applications ranging from forensics to social media. Although previous works reported performances that closely match human level, these solutions lack of human intuition that allows human beings to state the confidences of their predictions. While the human intuition subconsciously considers surrounding conditions or the lack of experience in a certain task, current algorithmic solutions tend to mispredict with high confidence scores. In this work, we propose a multi-algorithmic fusion approach for age and gender estimation that is further able to accurately state the model's prediction reliability. Our solution is based on stochastic forward passes through a dropout-reduced neural network ensemble. By utilizing multiple stochastic forward passes combined from the neural network ensemble, the centrality and dispersion of these predictions are used to derive a confidence statement about the prediction. Our experiments were conducted on the Adience benchmark. We showed that the proposed solution reached and exceeded state-of-the-art performance for the age and gender estimation task. Further, we demonstrated that the reliability statements of the predictions of our proposed solution captures challenging conditions and underrepresented training samples.
Exploring the Channels of Multiple Color Spaces for Age and Gender Estimation from Face Images
Authors: Fadi Boutros, Naser Damer, Philipp Terhörst, Florian Kirchbuchner, Arjan Kuijper (all Fraunhofer IGD)
Abstract: Soft biometrics identify certain traits of individuals based on their sampled biometric characteristics. The automatic identification of traits like age and gender provides valuable information in applications ranging from forensics to service personalization. Color images are stored within a color space containing different channels. Each channel represents a different portion of the information contained in the image, including these of soft biometric patterns. The analysis of the age and gender information in the different channels and different color spaces was not previously studied. This work discusses the soft biometric performances using these channels and analyzes the sample error overlap between all possible channels to successfully prove that different information is considered in the decision making from each channel. We also present a multi-channel selection protocols and fusion solution of the selected channels. Beside the analyzes of color spaces and their channels, our proposed multi-channel fusion solution extends beyond state-of-the-art performance in age estimation on the widely used Adience dataset.
Robust Face Authentication Based on Dynamic Quality-weighted Comparison of Visible and Thermal-to-visible Images to Visible Enrollments
Authors: Khawla Mallat (EURECOM, France), Naser Damer (Fraunhofer IGD), Fadi Boutros (Fraunhofer IGD), Jean-Luc Dugelay (EURECOM, France)
Abstract: We introduce, in this paper, a new scheme of score level fusion for face authentication from visible and thermal face data. This proposed scheme provides a fast and straightforward integration into existing face recognition systems and does not require recollection of enrollment data in thermal spectrum. In addition to be used as a possible countermeasure against spoofing, this paper investigates the potential role of thermal spectrum in improving face recognition performances when employed under adversarial acquisition conditions. We consider a context where individuals have been enrolled solely in visible spectrum, and their identity will be verified using 2 sets of probes: visible and thermal. We show that the optimal way to proceed is to synthesis a visible image from the thermal face in order to create a synthetic-visible probe; and then to fuse scores resulting from comparisons between visible gallery with both visible probe and synthetic-visible probe. The thermal-to-visible face synthesis is performed using a Cascaded Refinement Network (CRN) and face features were extracted and matched using LightCNN and Local Binary Patterns (LBP). The fusion procedure is performed through several quality measures computed on both visible and thermal-to-visible generated probes and compared to the visible gallery images.
FUSION 2019 is taking place in Ottawa, Canada from July 2 - 5, 2019.
Inormationen about FUSION 2019
show all news