News
Successful submissions of CRISP researchers to biometrics conference
Four publications by researchers at the Fraunhofer IGD were accepted at the 10th IEEE International Conference on Biometrics: Theory, Applications and Systems (BTAS). They will present them end of September in Tampas, Floriada. In addition, Dr. Naser Damer, Fraunhofer IGD, is co-organizer of the Special Session „Special Session on Generalizability and Adaptability in Biometrics (GAPinB)“. BTAS is one of the premier global conferences on biometric recognition and includes sensors, image and signal processing, pattern recognition, artificial intelligence, and statistics.
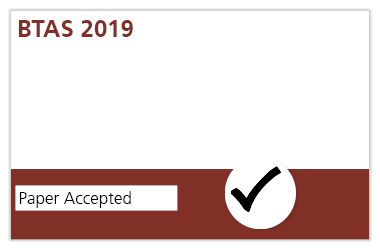
Accepted papers are:
Realistic Dreams: Cascaded Enhancement of GAN-generated Images with an Example in Face Morphing Attacks
Authors: Naser Damer, Fadi Boutros, Alexandra Moseguí Saladié, Florian Kirchbuchner, Arjan Kuijper
Abstract: The quality of images produced by generative adversarial networks (GAN) is commonly a trade-off between the model size, its training data needs, and the generation resolution. This trad-off is clear when applying GANs to issues like generating face morphing attacks, where the latent vector used by the generator is manipulated. In this paper, we propose an image enhancement solution designed to increase the quality and resolution of GAN-generated images. The solution is designed to require limited training data and be extendable to higher resolutions. We successfully apply our solution on GAN-based face morphing attacks. Beside the face recognition vulnerability and attack detectability analysis, we prove that the images enhanced by our solution are of higher visual and quantitative quality in comparison to unprocessed attacks and attack images enhanced by state-of-the-art super-resolution approaches.
Reliable Age and Gender Estimation from Face Images: Stating the Confidence of Model Predictions
Authors: Philipp Terhörst, Marco Huber, Jan Kolf, Ines Zelch, Naser Damer, Florian Kirchbuchner, Arjan Kuijper
Abstract: Automated age and gender estimation became of great importance for many potential applications ranging from forensics to social media. Although previous works reported high increased performances, these solutions tend to mispredict under challenging conditions or when the trained model faces a sample that was underrepresented in the training data. In this work, we propose an age and gender estimation model, as well as a novel reliability measure to quantify the confidence of the model’s prediction. Our solution is based on stochastic forward passes through dropout-reduced neural networks that were theoretically proven to approximate Gaussian processes. By utilizing multiple stochastic forward passes, the centrality and dispersion of these predictions are used to derive a confidence statement about the prediction. Experiments were conducted on the Adience benchmark. We showed that the proposed solution reached and exceeded state-of-the-art performance. Further, we demonstrated that the proposed reliability measure correlates with the prediction performance and thus, is highly successful in quantifying the prediction reliability.
On Learning Joint Multi-biometric Representations by Deep Fusion
Authors: Naser Damer, Kristiyan Dimitrov, Florian Kirchbuchner, Arjan Kuijper
Abstract: Multi-biometrics combines different biometric sources to enhance recognition, template protection, and indexing performances. One of the main challenges here is the need for joint discriminant feature representation of multi-biometric data. This is typically achieved by feature-level fusion, imposing limitations on the combinations of biometric characteristics and algorithms. Including multiple imaging sources within deep-learning networks was generally limited to multiple sources of images of the same physical object, e.g., multi-spectral object detection. Previous biometrics works were limited to use deep-learning to extract representations of single biometric characteristics. In contrast to that, our work studies creating representations of one identity by sampling different physical objects,i.e. biometric characteristics. We adapted three architectures successfully to produce and discuss jointly learned representations for different levels of correlated data, modalities, instances, and presentations. Our evaluation proved the applicability of jointly learning biometric representations, especially when the data correlation is low.
On the Generalization of Detecting Face Morphing Attacks as Anomalies: Novelty vs. Outlier Detection
Authors: Naser Damer, Jonas Henry Grebe, Steffen Zienert, Florian Kirchbuchner, Arjan Kuijper
Abstract: Face morphing attacks are verifiable to multiple identities, leading to faulty identity links. Recent works have studied the face morphing attack detection performance over variations in morphing approaches, pointing out low generalization. This work studies detecting these attacks as anomalies and discusses the performance and generalization over different morphing types. We also analyze the accuracy and generalization effect of including different amounts of attack contamination in the anomaly training data (novelty vs. outlier). This is performed with two baseline 2-class classifiers, two approaches for anomaly detection, two image feature extractions, two morphing types, and variations in contamination levels and tolerated training errors. The results points out the relative lower performance, but higher generalization ability, of anomaly detection in comparison to 2-class classifiers, along with the benefits of contaminating the anomaly training data.
BTAS 2019 will be held in Tampa, Florida, on September 23 - 26.
Information about the conference
show all news