DeCNeC: Detecting CSAM Without the Need for CSAM Training Data
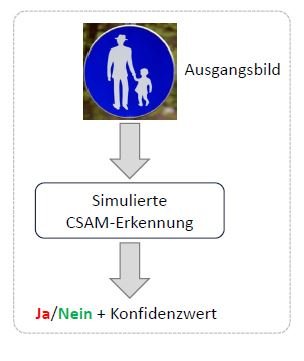
The detection of child sexual abuse material (CSAM) presents a significant challenge for law enforcement agencies worldwide. While known CSAM, such as material seized during investigations, can be identified using hash-matching techniques (e.g., perceptual hashes like PhotoDNA), the detection of unknown CSAM requires advanced methods - particularly those based on artificial intelligence (AI). However, the use of CSAM training data in AI is highly problematic due to its severely illegal nature (see StGB §184b+c), especially for research institutions.
This is where the DeCNeC project comes in, focusing on the detection of unknown CSAM without relying on CSAM data. The project explores and develops innovative approaches in the field of computer vision. The core idea involves combining separate concepts to enable CSAM detection. Specifically, classifiers are trained separately on adult pornographic scenes and on harmless images of children in everyday situations. These classifiers are then fused to identify potential overlaps (and thus CSAM). Additionally, DeCNeC is focusing on research on image enhancement techniques to effectively work with low-quality images that may arise in practice. In parallel, the project develops solutions to address challenges such as problematic pose representations (e.g., as defined by the COPINE scale).
In close collaboration with law enforcement agencies, DeCNeC aims to develop ethical and practical detection methods that can support investigative work with precision and efficiency.